Integrating abduction, visualization, and explanation as a data architecture for Artificial Intelligence
Randy Goebel, University of Alberta, Canada
Workshop MAKE-Explainable AI, Thursday 30.08.2018, 9:30-11:00
Abstract: The integration of abduction, visualization, and explanation provides a fundamental data architecture for artificial intelligence (AI).
Abduction has been described as constrained induction, which provides the basis for using what is already known to focus the synthesis — both creation and adjustment — of scientific theories. Visualization is inherently about how to appropriately present information for drawing inferences by the human visual system. And explanation is at the heart of the scientific process, which, in all its forms, is about connecting theories and evidence across a spectrum from exposing relationships between observation and theory, all the way to exposing causality.
We will attempt to create coherence around these three foundational ideas, show how they can be related in both theory and practice, by use of examples of multi-level representations that can exploit AI and machine learning for both humans and machines.
R.G. (Randy) Goebel is Professor of Computing Science at the University of Alberta, in Edmonton, Alberta, Canada, and concurrently holds the positions of Associate Vice President Research, and Associate Vice President Academic. He is also co-founder and principle investigator in the Alberta Innovates Centre for Machine Learning. He holds B.Sc., M.Sc. and Ph.D. degrees in computer science from the University of Regina, Alberta, and British Columbia, and has held faculty appointments at the University of Waterloo, University of Tokyo, Multimedia University (Malaysia), Hokkaido University, and has worked at a variety of research institutes around the world, including DFKI (Germany), NICTA (Australia), and NII (Tokyo), was most recently Chief Scientist at Alberta Innovates Technology Futures. His research interests include applications of machine learning to systems biology, visualization, and web mining, as well as work on natural language processing, web semantics, and belief revision. He has experience working on industrial research projects in scheduling, optimization, and natural language technology applications.
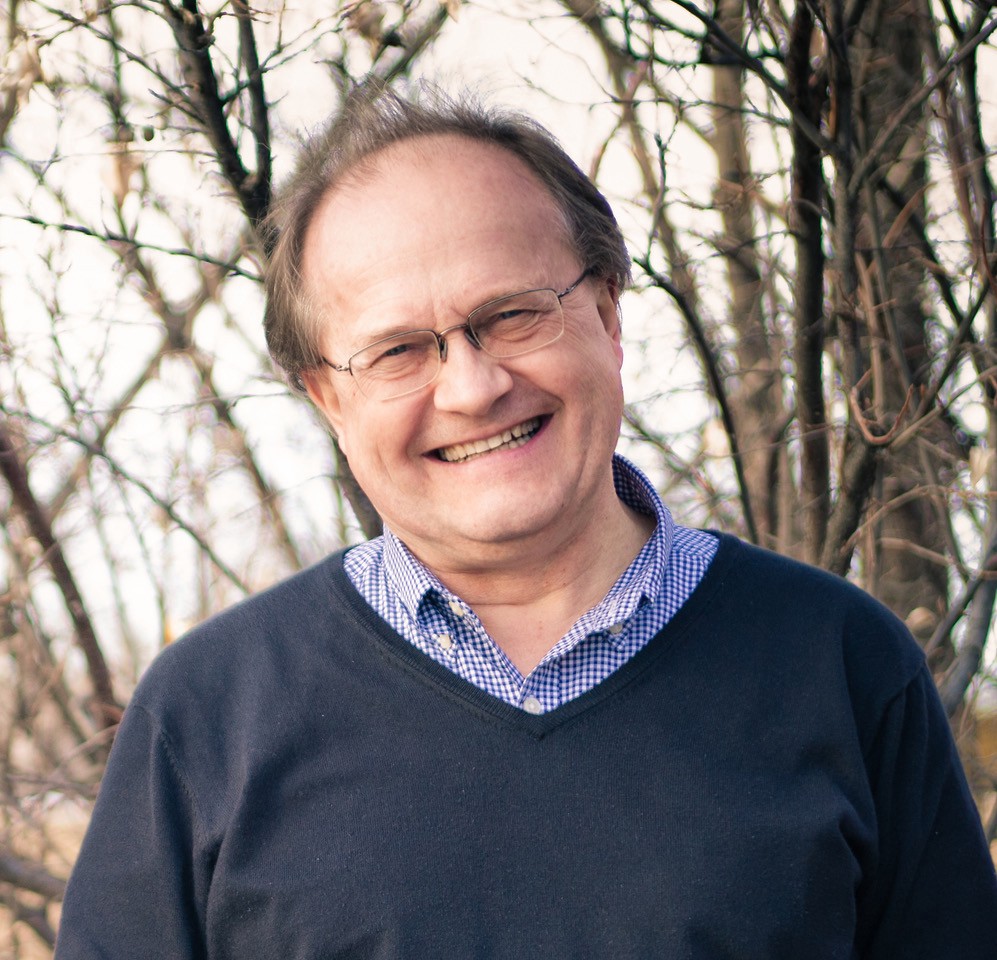